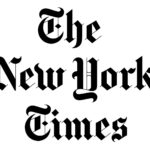
Article on the New York Times
Our latest paper on the connection between attitude networks and social identity was discussed in this article from the New…
– Social Complexity –
Seamless Theme Just Pretty, made by Altervista
Create a website and earn with Altervista - Disclaimer - Report Abuse